Spatial Proteomics Sheds Light on Breast Cancer Progression, Gives Hope for Prognostic Test
To understand signs of progression to invasive breast cancer
It’s a decades-old mystery among oncologists: why do some women diagnosed with non-invasive breast tumors progress to invasive breast cancer while others do not? Understanding this — and being able to predict accurately which women fall into which category — would allow clinicians to provide more aggressive treatment to those who need it most, and spare many patients painful and debilitating treatment that is unnecessary.
Recently, scientists at Stanford University and other institutions collaborated to answer this question using a unique combination of valuable tools: Washington University’s archival breast tissue collection, clinically annotated with more than a decade of cancer progression data; multiplexed ion beam imaging (MIBI™) technology for high-dimensional detection of spatially resolved proteins; and a machine learning approach to make sense of the tremendous amount of data generated.
Based on their work, the team discovered highly predictive elements associated with progression or non-progression in women diagnosed with ductal carcinoma in situ (DCIS). Their efforts have paved the way for future development of a prognostic test that could be a huge leap forward for the 60,000 women diagnosed with DCIS each year in the U.S. alone.
Characterizing DCIS progression
“In light of this uncertainty, clinical management has trended towards treating all patients presumptively as progressors with surgery, radiation therapy, and pharmacological interventions that carry risks for therapy-related adverse events,” the authors report. They posited that spatial proteomics data, which can produce more actionable data related to phenotype, might yield new answers and possibly even help guide treatment decisions.
The team turned to the Washington University Resource Archival Human Breast Tissue cohort, a treasure trove of FFPE samples that includes patients diagnosed with DCIS and records of their outcomes over 11 years, including which patients progressed to invasive breast cancer. Because DCIS is diagnosed based on its hallmark structure — a very specific organization of tumor, stromal, and myoepithelial cells — the scientists were eager to deploy MIBI spatial proteomics technology to resolve the precise cell location, composition and function using 37 proteins of interest.
They used this approach to characterize 122 samples featuring normal breast, DCIS, or invasive breast cancer. Then they implemented machine learning tools to identify and map 16 different cell populations and their varied spatial parameters across the samples. Overall, they measured more than 1,000 features in each sample and incorporated these into a classifier to determine which ones, if any, could be clearly linked to progression patterns.

How MIBI technology made a difference
“With so many diverse cell types in the stroma, myoepithelium, and immune component, the multiplex capability of MIBI technology was one of its most useful advantages over other technologies,” Risom says. “Typically the multiplex capability of approaches like multiplex immunofluorescence falls short of being able to look at all those factors at once and show us their spatial organization and localization.”
The fact that it can be used with archival FFPE samples was also critical. “That allowed us to look at this very special, very powerful patient cohort,” Risom adds.
A unique feature of DCIS made its spatial organization of particular interest to scientists: its tumor cells are held at bay by a very thin boundary of myoepithelial cells. When that boundary fails, it gives cancer the opportunity to invade surrounding breast tissue. But studying these cells has been a major challenge. “There are so few of them and they’re stretched so thin,” Risom says. Using a pixel-based clustering approach with MIBI technology, his team was able to profile the myoepithelium as a layer of tissue. “We were able to isolate the myoepithelial layer and assess its thickness and continuity around the tumor based on how many nuclei or cells are within a certain amount of space,” Risom says.
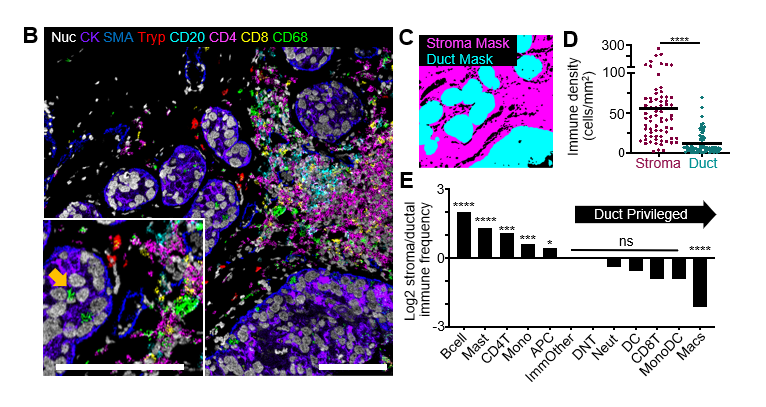
Key discoveries and next steps
One of those defining features was an E-cadherin high myoepithelial phenotype that appears to be highly enriched in patients whose DCIS later progressed to invasive disease. “It was really prominent, and it took the resolution and multiplexing powers of MIBI to be able to isolate that thin membrane and spot the expression state,” Risom says.
Other features, many of them structural, were also associated with progression. All of these candidates will be followed up in future studies in the Angelo lab to better understand their link to disease state. In addition, Risom anticipates that the team will work to highlight the core features needed to predict which women are at highest and lowest risk of progression. “We want to boil this down to the smallest set of useful features to develop a clinical prognostic test,” he says. “If we can identify a low-risk population that doesn’t need surgery or radiation, that could really improve quality of life for those patients.”
This work was done as part of the Human Tumor Atlas Network. Once released the full results will be available there.